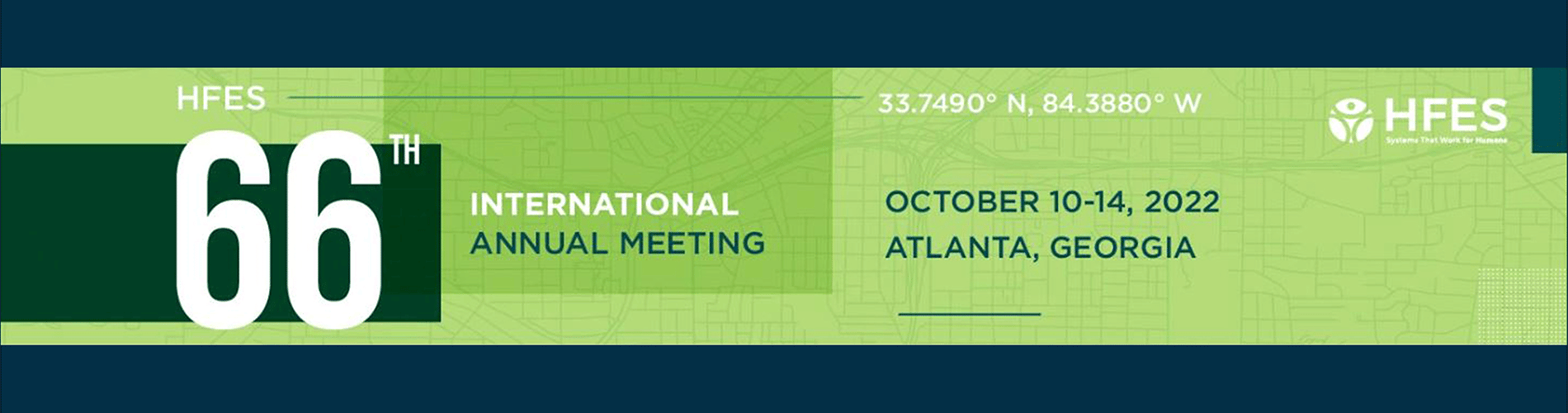
Aptimist Isabel Erikson Co-Author Recipient of HFES 2022 Alphonse Chapanis Student Paper Award
Award recognizes students for outstanding human factors research
Aptima congratulates associate research engineer Isabel Erikson as a co-author recipient of the 2022 Alphonse Chapanis Award at the Human Factors and Ergonomics Society Annual Meeting (Oct 10-14, Atlanta GA).
Mengyao Li, Isabel, Ernest V. Cross, and John D. Lee were recognized for “Estimating Trust in Conversational Agent with Lexical and Acoustic Features,” which Isabel co-authored while a student at University of Wisconsin–Madison.
Established in 1969, the Alphonse Chapanis award is presented to a student or students for outstanding human factors research conducted while enrolled in an appropriate academic program and presented as a paper or poster at the HFES Annual Meeting. Students apply for this award by submitting an application form along with their full proceedings papers to the award committee chair. Short abstracts are not eligible for this award. The award application form is made available to accepted authors in May and a cash award of $2,000 along with a certificate are provided to the winner(s).
For a courtesy copy of this paper, please contact aptima_info@aptima.com.
Abstract
As NASA moves to long-duration space exploration operations, there is an increasing need for human-agent cooperation that requires real-time trust estimation by virtual agents. Our objective was to estimate trust using conversational data, including lexical and acoustic features, with machine learning. A 2 (reliability) × 2 (cycles) × 3 (events) within-subject study was designed to provoke various levels of trust. Participants had trust-related conversations with a conversational agent at the end of each event. To estimate trust, subjective trust ratings were predicted using machine learning models trained on three types of conversational features (i.e., lexical, acoustic, and combined). Results showed that a random forest model, trained on the combined lexical and acoustic features, best predicts trust in the conversational agent (R2 adj = 0.67). Comparing models, we showed that trust is not only reflected in lexical cues but also acoustic cues. These results show the possibility of using conversational data to measure trust unobtrusively and dynamically.