Our goals
Aptima’s most innovative products and services are the result of innovative technical solutions
Grouped under the Human-Centered Innovations™ brand, the resulting novel products and services allow Aptima to uniquely provide services to our customers
Human-Centered Innovations reflect Aptima’s products and services directed at capturing and building on innovative technologies developed by the Aptima team. These technologies are directed at business management solutions, engineering, and R&D solutions, as well as software products and services. Typically protected by a core of patents or other intellectual property, these Human-Centered Innovations products and services can easily be used to help solve the needs of our clients.
Examples of business and technical areas where these Human-Centered Innovations have been directed in the past include:
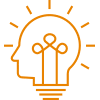
Human Factors Engineering
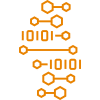
Cognitive Sciences
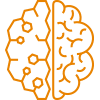
Artificial Intelligence
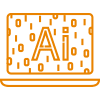
Computer-Based Training and Simulations
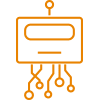
Big Data Analysis
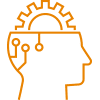
Network/Data Pattern Matching
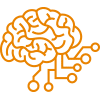
Social Networking and Analytics
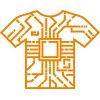
Training and Decision Support Systems
Examples of some of the technologies included in Aptima’s Human-Centered Innovations products and services include the patents listed below. If you are interested in more information regarding Aptima’s Human-Centered Innovations products or services, please engage us at Patent@Aptima.com or ask for the Intellectual Property group at 781-935-3966.
Patents
Aptima’s Patents
Patents are listed in descending order, with most recent patents at the top.
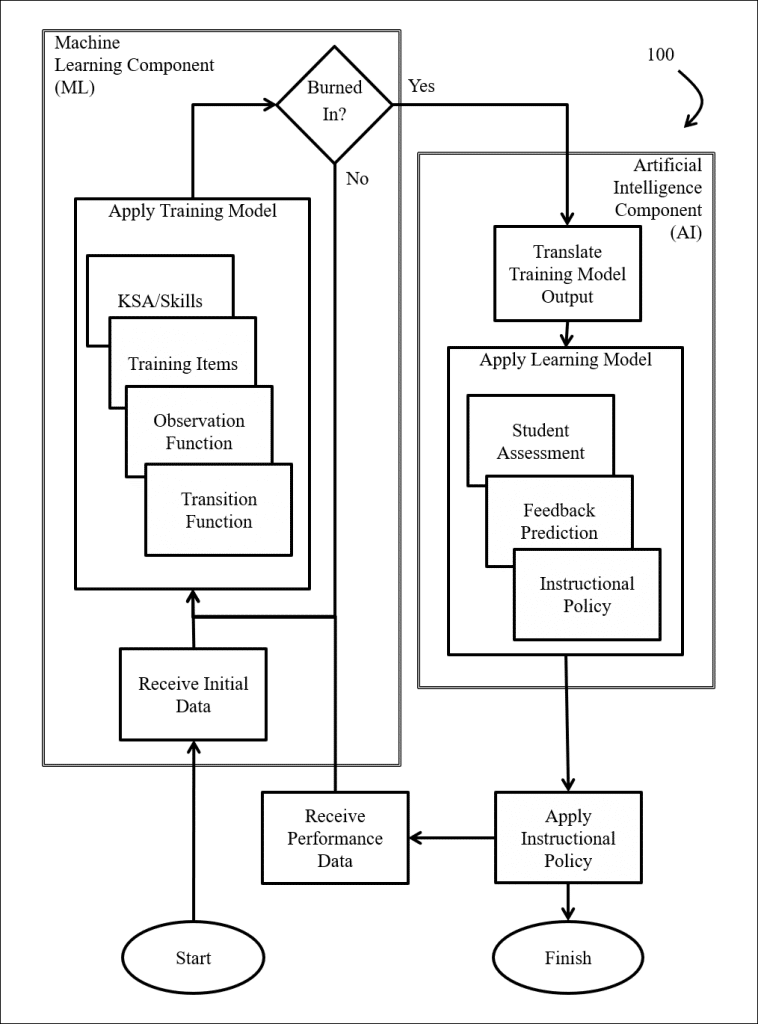
Systems and methods for automated learning
Title: Systems and methods for automated learning
Issue Date: 2/4/2020
Inventors: Carlin
Abstract: A computer implemented systems and methods for determining an action for a user within a learning domain are disclosed, some embodiments of the methods comprise defining an initial learning model of a learning domain, determining an initial user state of the user, determining an initial user action from at least one learning domain action with the initial learning model, receiving a user observation of the user after the user executes the initial user action, determining an updated user state with the initial learning model given the updated user observation and determining a subsequent user action from the at least one learning domain action. Some embodiments utilize a Partially Observable Markov Model (POMDP) as the learning model.
Systems and methods to provide training guidance
Issue Date: 10/8/2019
Inventors: Swanson, Reynolds, Garrity, Poeppelman, Keeney, Carlin, Dumond, Marc
Abstract: Systems and methods to provide a training solution for a trainee are disclosed. In some embodiments the method comprises receiving a training requirement comprising a training outcome and a training configuration wherein the training configuration defines a trainee state, determining a training environment based on a relevancy function of the training environment to the training outcome, determining a training content based on a relationship function of the training content to the trainee state and determining a training solution comprising the training environment and the training content. In some embodiments, the relationship function comprises a POMDP model and the relevancy function comprises a best fit curve.
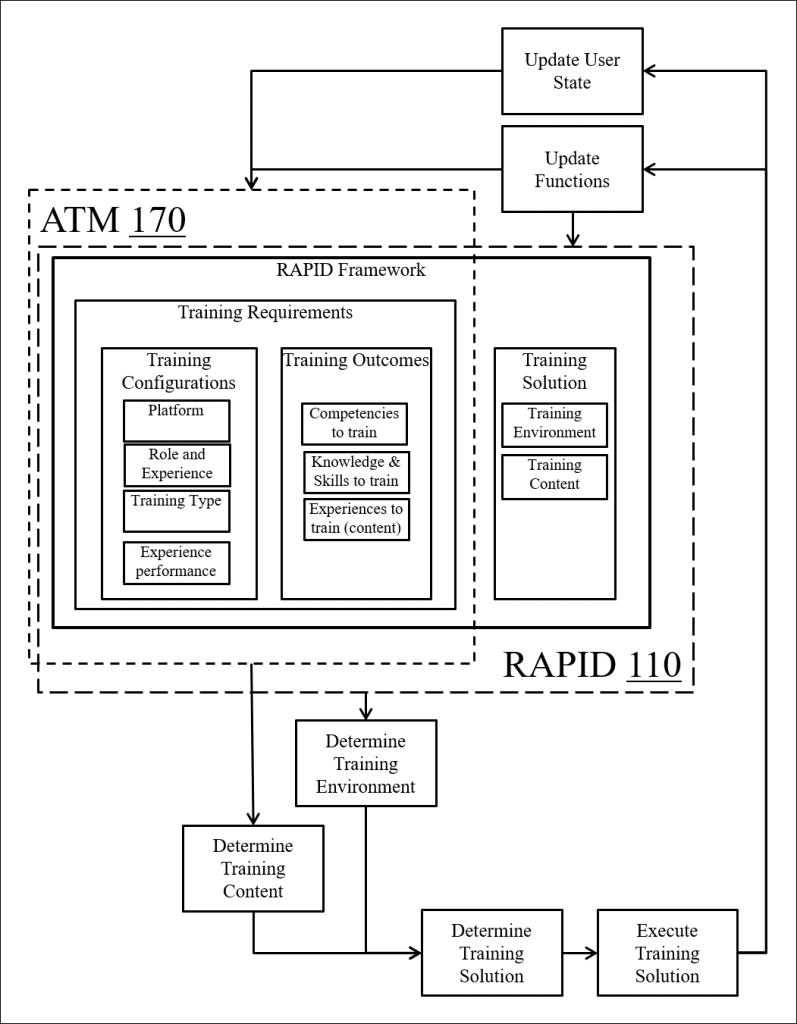
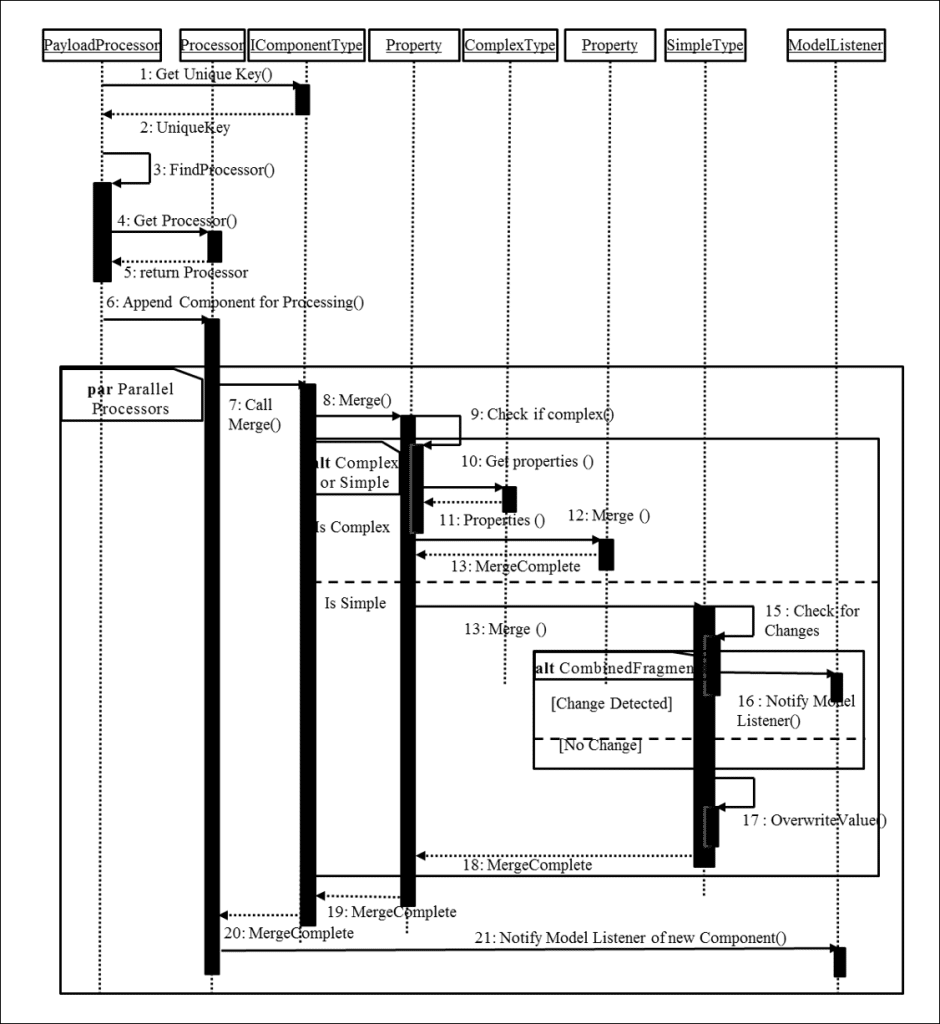
Systems and methods to measure performance – large entity
Issue Date: 10/1/2019
Inventors: Tolland, Zuzack
Abstract: A performance measurement system is provided utilizing an architecture configured to efficiently merge and monitor different types of performance data. Connectors are provided to receive and translate different types of performance data from different sources. The performance data is translated into and stored in a common data model format. In some embodiments, key attributes are defined for each of the performance data sources that uniquely characterizes each relevant performance data so that is can be parsed into separate processing streams to increase system performance. The key attributes also act as cues to organize the performance data as it is being merged so that it can be accessed without requiring a specific source data linkage. Using model listeners, determinations can be quickly made regarding when performance data is changed to reduce calculations necessary to determine measure values. Some embodiments merge different types of performance data in real-time.